AI-Powered Cryptoeconomic Models for Optimizing Blockchain Incentive Structures
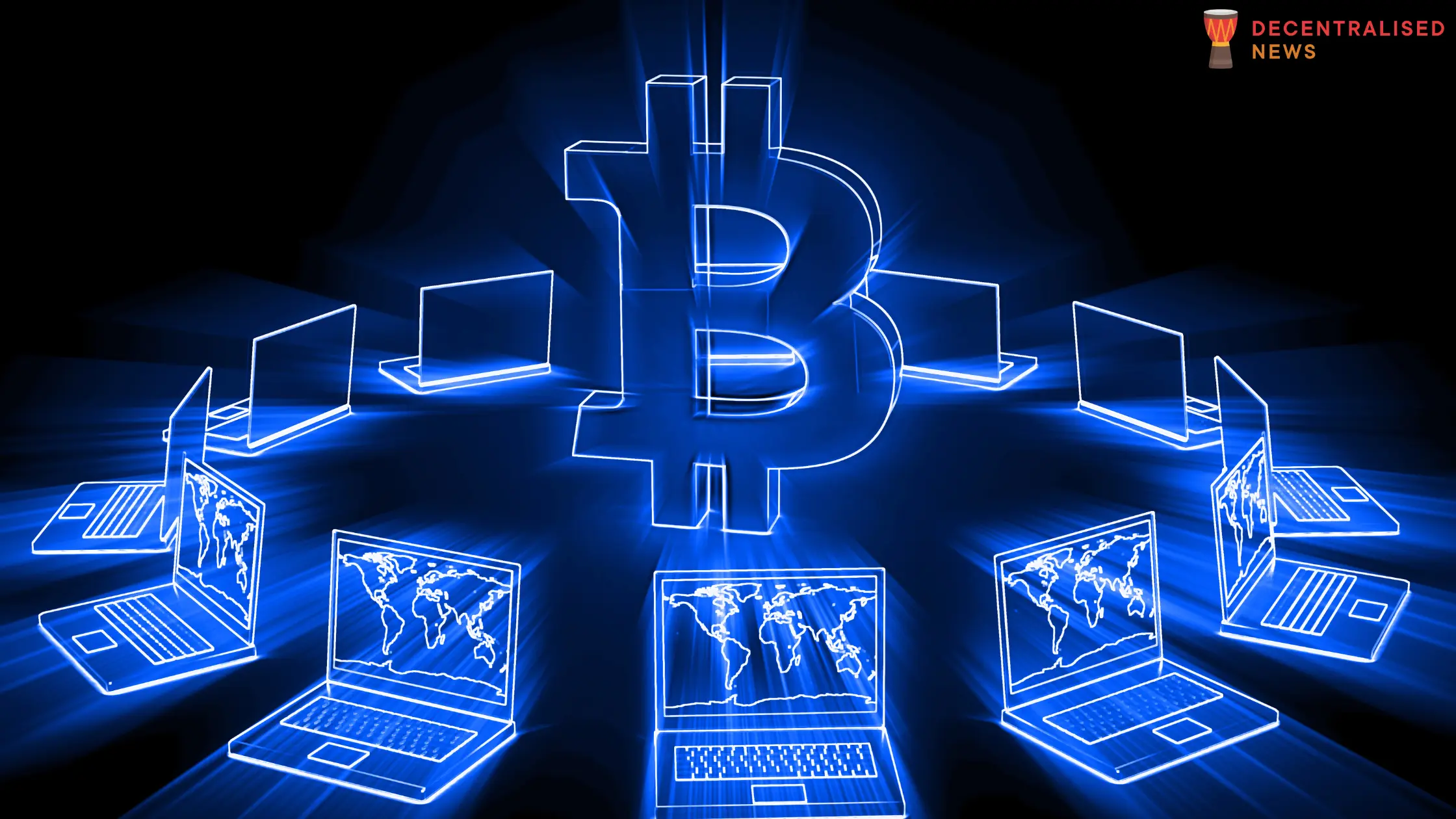
Cryptoeconomic models are the foundation of blockchain networks, dictating how participants are incentivized to maintain and secure the network. With the integration of artificial intelligence (AI), these models can be optimized to create more efficient and resilient incentive structures. This article explores how AI can enhance cryptoeconomic models, the benefits of AI-driven incentive mechanisms, and potential challenges and future directions in this emerging field.
Understanding Cryptoeconomic Models
Cryptoeconomic models combine cryptography and economic incentives to ensure the security, functionality, and decentralization of blockchain networks. These models define how participants (nodes, miners, stakers, etc.) are rewarded for their contributions and penalized for malicious behavior.
Proof of Work (PoW): In PoW, miners compete to solve complex mathematical puzzles. The first to solve the puzzle adds a new block to the blockchain and receives a reward in the form of cryptocurrency.
Proof of Stake (PoS): In PoS, validators are chosen to create new blocks and validate transactions based on the number of coins they hold and are willing to “stake” as collateral.
Delegated Proof of Stake (DPoS): In DPoS, coin holders vote to elect a small number of delegates who are responsible for validating transactions and maintaining the blockchain.
AI’s Role in Enhancing Cryptoeconomic Models
AI can significantly improve the design and optimization of cryptoeconomic models by analyzing vast amounts of data, predicting participant behavior, and dynamically adjusting incentive mechanisms.
AI can predict the behavior of network participants based on historical data, enabling more accurate modeling of incentive structures. For example, AI can analyze past mining or staking patterns to predict future behaviors, allowing the network to adjust rewards and penalties accordingly.
AI can enable real-time adjustments to incentive structures based on network conditions and participant behavior, ensuring optimal network performance and security. For example, If AI detects an increase in malicious activity, it can temporarily increase penalties or rewards to incentivize honest behavior and enhance network security.
AI can analyze the behavior of participants to identify patterns and potential risks, such as collusion or attacks, and adjust the cryptoeconomic model to mitigate these risks. For example, AI can detect patterns of voting collusion in a DPoS system and adjust the weight of votes or implement additional checks to ensure fair representation.
AI can apply advanced game theory to optimize incentive structures, ensuring that participants are motivated to act in the best interest of the network. For example, AI can model various scenarios and strategies to determine the most effective reward and penalty structures that discourage selfish behaviour and promote collaboration.
Benefits of AI-Enhanced Incentive Structures
AI-driven models can optimize resource allocation and network performance, reducing the computational and energy costs associated with consensus mechanisms like PoW.
By dynamically adjusting incentives and detecting malicious behavior, AI can enhance the security and resilience of blockchain networks against attacks.
AI allows cryptoeconomic models to adapt to changing network conditions and participant behaviors in real-time, ensuring continuous optimization and stability.
AI can identify and mitigate unfair practices, such as vote manipulation or mining centralization, promoting a more equitable distribution of rewards.
AI-driven models can improve the scalability of blockchain networks by optimizing the validation process and reducing bottlenecks.
Challenges and Considerations
Implementing AI-enhanced cryptoeconomic models can be complex and require significant expertise in both AI and blockchain technologies.
The effectiveness of AI depends on the quality and accuracy of the data it processes. Inaccurate or incomplete data can lead to flawed models and suboptimal decisions.
Participants need to trust that the AI-driven models are fair and transparent. Ensuring transparency in AI algorithms and their decision-making processes is crucial.
AI-enhanced cryptoeconomic models must comply with regulatory requirements, which can vary by jurisdiction. Ensuring legal compliance is essential to avoid regulatory issues.
AI models must be designed and implemented ethically, ensuring they do not perpetuate biases or unfair practices.
Future Directions
The integration of AI into cryptoeconomic models is an emerging field with significant potential for innovation. Future research and development may focus on:
Developing more sophisticated machine learning algorithms to better predict participant behavior and optimize incentive structures.
Ensuring AI-driven cryptoeconomic models can operate across multiple blockchain networks, enhancing interoperability and collaboration.
Exploring decentralized AI solutions that operate within the blockchain ecosystem, reducing reliance on centralized AI providers.
Working with regulators to develop frameworks that support the use of AI in cryptoeconomic models while ensuring compliance and protecting participants.
Encouraging collaboration between AI researchers, blockchain developers, and economists to create more robust and effective cryptoeconomic models.
Key Takeaways
AI-enhanced cryptoeconomic models offer a promising avenue for optimizing incentive structures in blockchain networks. By leveraging predictive analytics, dynamic adjustments, behavior analysis, and game theory, AI can create more efficient, secure, and fair cryptoeconomic systems. While challenges remain, the benefits of integrating AI into cryptoeconomic models are significant, paving the way for more resilient and scalable blockchain networks.
As research and development in this field continue to advance, AI-driven cryptoeconomic models are likely to play a crucial role in the future of blockchain technology.